In conjunction with 26th International Conference On Pattern Recognition (ICPR 2022). Please register on the ICPR website to participate.
Aims and scope
As one of the flagship events of the International Association for Pattern Recognition, Pattern Recognition in Remote Sensing Workshop brings together researchers from both pattern recognition and remote sensing, with emphasis on the application of pattern recognition methods to remotely sensed data. The steady progress in the development of new remote sensing devices and remote sensing technology has led to ever-increasing data and new challenges. Thus, a need arises to apply the latest methods of machine learning and pattern recognition for automated analysis of this kind of data. This workshop will provide an ideal forum for spreading and exchanging the latest experiences of international researchers.
Topics
Researchers are welcome to submit papers in the following areas or related topics:
- Semantic classification and parameter estimation from hyperspectral and multispectral images
- Extraction, selection, learning, and reduction of features
- Deep learning for Earth observation data
- Clustering
- Active and transfer learning
- Multi-modal and multi-temporal analysis
- Image analysis of SAR and airborne thermal data
- Recognition of man-made objects from aerial and space platforms
- Novel pattern recognition tasks in remote sensing applications
- Explainable and interpretable machine learning
- Hybrid models (physics+machine learning)
- Benchmark datasets
- Technical reviews on related topics
Program: August 21, 2022
9:00 (Montréal time, EDT, GMT-4) Welcome
Ribana Roscher (University of Bonn), Sylvain Lobry (Université de Paris)
9:15 – 10:15 Keynote
The Scarcity of Labels for Satellite Images: Opportunity and Challenges of Multi-source Geo-tagged Data (online)
Claudia Paris
10:15 – 10:30 Break
10:30 – 11:30 Session 1: Learning with Multiple Models and Inputs
Physics-Informed Neural Networks For Solar Wind Prediction (online)
Rob Johnson (Utah State University); Soukaina Filali Boubrahimi (Utah State University)*; Shah Muhammad Hamdi (New Mexico State University); Omar Bahri (Utah State University)
Less Labels, More Modalities: A Self-Training Framework to Reuse Pretrained Networks
Jean-Christophe Burnel (Université de Bretagne Sud / IRISA)*; Sébastien Lefèvre (Université de Bretagne Sud / IRISA); Luc Courtrai (IRISA UBS)
Feature Transformation for Cross-domain Few-shot Remote Sensing Scene Classification (online)
Qiaoling Chen (South China Agricultural University); Zhihao Chen (South China Agricultural University); Wei Luo (South China Agricultural University)*
11:30 – 13:00 Lunch break
13:00 – 14:00 Keynote
Earth Observation Data for Geospatial Artificial Intelligence (online)
Elif Sertel (Istanbul Technical University)
14:00 – 14:15 Break
14:15 – 15:15 Session 2: Analyzing and Interpreting SAR Imagery
A Novel Methodology for High Resolution Sea Ice Motion Estimation
Kelsey P Kaplan (University of Delaware)*
Deep Learning-based Sea Ice Lead Detection from WorldView and Sentinel SAR Imagery
Rohit Venkata Sai Dulam (University of Delaware)*; Kelsey P Kaplan (University of Delaware); Chandra Kambhamettu (University of Delaware)
Uncertainty Analysis of Sea Ice and Open Water Classification on SAR Imagery using a Bayesian CNN
Xinwei Chen (University of Waterloo)*; K Andrea Scott (University of Waterloo); David A Clausi (University of Waterloo)
15:15 – 15:30 Break
15:30 – 16:30 Session 3: Semantic Segmentation and Detection
A Two-Stage Road Segmentation Approach for Remote Sensing Images (online)
Tianyu Li (Purdue University)*; Mary Comer (Purdue University); Josiane Zerubia (n/a)
YUTO Tree-5000: A Large-scale Airborne LiDAR Data for Single Tree Detection
Connie Ko (York University)*; Yeonjeong Jeong (York University); Hyungju Lee (York University); Gunho Sohn (York University)
Spatial Layout Consistency for 3D Semantic Segmentation
Maryam Jameela (York University)*; Gunho Sohn (York University)
16:30 Closing Remarks
Keynote Speakers
Elif Sertel
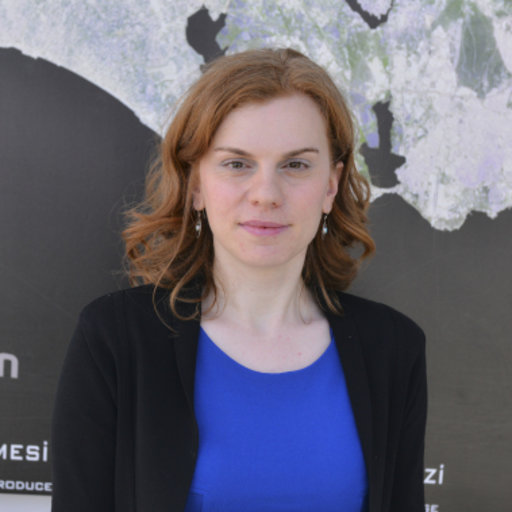
Elif Sertel is a Professor (since 2016) at the Geomatics Engineering Department of Istanbul Technical University. She conducted her PhD dissertation work at Rutgers University, the USA, as a Fulbright Scholar and investigated the impacts of land cover change on regional climate. She was a post-doctoral researcher at Rutgers University in 2008 and explored the effects of water table dynamics on the regional climate.
She held the Director position at Istanbul Technical University – Center for Satellite Communications and Remote Sensing (ITU-CSCRS) between 2012 and 2021, where she was the Vice-Director between 2009 and 2012.
Her research focuses on the application of geospatial technologies, namely Remote Sensing and Geographic Information Systems (GIS), to solve environmental issues, generate decision support systems, understand the interactions between the land surface and climate and the impacts of humans on climate, primarily due to the land cover change caused by urbanization. For the last five years, she has mostly worked on deep learning, geospatial data analytics, and environmental problems such as soil salinity and droughts. She has extensively used geostatistical and spatial methods for the quantitative impact analyses of multidisciplinary applications, specifically in agriculture, forestry, and urban planning. Recently, she has also been researching the application of GeoAI to spatial challenges in the Digital Humanities.
Prof. Sertel received the Outstanding Young Scientist Award from the Turkish Academy of Sciences in 2017 (TÜBA-GEBİP) and the TUBITAK Incentive Award in Engineering from TUBITAK (The Scientific & Research Council of Turkey) in 2021; the first scientist in Turkey to receive both awards in her field.
She is in the Editorial Board of “Geocarto International” and “International Journal of Digital Earth” journals. She is acting as a Guest Editor for the special issue “Advances in Large-Scale Computer Vision for Earth Observation Data Analytics” in the “International Journal of Applied Earth Observation and Geoinformation”
Title: Earth Observation Data for Geospatial Artificial Intelligence
Abstract:
The number of Earth Observation (EO) satellites in orbit has grown significantly with the contribution of the NASA and ESA Earth Observation programs and numerous commercial initiatives. As a result, there is a substantial increase in satellite image data volume and variety. Cloud-based data provision and processing platforms have facilitated access to an enormous amount of high-resolution Remote Sensing (RS) images and opened a new era in Geospatial Artificial Intelligence (GeoAI)-based RS research.
This talk will provide different GeoAI methods implemented to EO data to support geospatial analytics processes. The first application will be on RS image super-resolution (SR). The spatial and spectral resolution of EO satellites are generally not at the desired high level due to the limitations on optic and sensor technology and high costs; therefore, it is crucial to develop software-based algorithms to improve the spatial and spectral quality of the satellite images, which emphasize the importance of SR. Different deep learning (DL) based methods for Single-frame and Multi-frame RS image resolution will be comprehensively explained. The second application will be geospatial object detection, in which airplane detection will be focused on by providing results of various YOLO architectures. The third GeoAI application will be on geospatial object segmentation, in which a new building detection data set will be presented and results of the comparison of different DL architectures for the accurate extraction of buildings for this new data set and widely used Massachusetts and INRIA data sets will be discussed. The last application will be on Land Use/Land Cover segmentation from high-resolution multi-sensor data, in which results of multi-modal and multi-task segmentation to reliably map extensive LULCC classes will be presented. Finally, future directions and insights on GeoAI data sets and methods for different applications will be discussed.
Claudia Paris
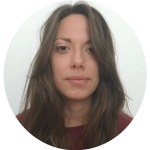
Claudia Paris is Assistant Professor at the University of Twente, Faculty ITC, Department of Natural Resources, Enschede, the Netherlands. Her main research interests are in remote sensing image processing, signal processing and pattern recognition, with a focus on classification and fusion of multisource remote sensing data, multitemporal image analysis, domain adaptation methods and deep learning models. She has been conducting research on these topics in the framework of national and international projects. She has been guest editor of the special issue “Advanced Remote Sensing Data Analysis for Sustainable Development” of the IEEE Journal of Selected Topics in Applied Earth Observations and Remote Sensing. She is a member of the scientific and programme committee of the IEEE International Geoscience and Remote Sensing Symposium (IGARSS) and the SPIE International Symposium on Remote Conferences, respectively, and is also a referee for several international journals. Dr Paris won the prestigious Symposium Prize Paper Award (SPPA) at the International Symposium on Geoscience and Remote Sensing 2016 (Beijing, China, 2016) and the International Symposium on Geoscience and Remote Sensing 2017 (Fort Worth, Texas, USA, 2017).
Title: The scarcity of labels for satellite images: opportunity and challenges of multi-source geo-tagged data
Abstract:
Recent advances in Earth observation data and artificial intelligence are paving the way for extremely detailed, near-daily environmental monitoring at very high spatial resolution. This possibility, however, is limited by the need for huge, constantly updated annotated data sets, collected on a large scale and customized for the environmental analyses under investigation. However, the recent abundance of real-time geospatial data continuously collected by social sensors (e.g. social media platforms), crowdsourced data (e.g. openstreetmaps) and the increasing production of global thematic maps are changing the paradigm of reference data collection. Although extremely valuable, these data are not 100% reliable, thus introducing in the reference database a degree of uncertainty that must be taken into account. This talk will provide an overview of the limitations, challenges and opportunities offered by all these multi-source geo-tagged datasets that can relieve the labeled data scarcity bottleneck.
Programm Committee
Alper Yilmaz, Ohio State University, USA
Benjamin Lucas, University of Colorado Boulder, USA
Bertrand Le Saux, European Space Agency (ESA), Italy
Camille Kurtz, University of Paris, France
Cem Ünsalan, Marmara Üniversitesi, Turkey
Clément Rambour, CNAM, France
David Clausi, University of Waterloo, Canada
Diego Marcos, Wageningen University, Netherlands
Dino Ienco, INRAE, France
Jenny Q. Du, Mississippi State University, USA
Eckart Michaelsen, Fraunhofer-IOSB, Germany
Emanuele Dalsasso, Telecom Paris, France
Fabio Dell’Acqua, University of Pavia, Italy
Flora Weissgerber, ONERA, France
Florence Tupin, Telecom Paris, France
Franz Rottensteiner, Leibniz Universitat Hannover, Germany
Gunho Sohn, York University, Canada
Helmut Mayer, Bundeswehr University Munich, Germany
Jan Dirk Wegner, University of Zurich, Switzerland
Jocelyn Chanussot, Grenoble Institute of Technology, France
John Kerekes, Rochester Institute of Technology, USA
Lloyd Hughes, EPFL, Switzerland
Loic Landrieu, IGN, France
Lorenzo Bruzzone, University of Trento, Italy
Martin Weinmann, Karlsruhe Institute of Technology, Germany
Michael Schmitt, Bundeswehr University Munich, Germany
Michele Volpi, Swiss Data Science Center, ETH Zurich, Switzerland
Nathan Jacobs, Washington University in St. Louis, USA
Nicolas Audebert, CNAM, France
Paolo Gamba, University of Pavia, Italy
Sébastien Lefèvre, Université de Bretagne Sud / IRISA, France
Selim Aksoy, Bilkent University, Turkey
Xuezhi Yang, Hefei University of Technology, China
Sponsors